Data is perhaps the most important resource of businesses in the current society given the technological advancement. The effectiveness of the operations of an organization as well as the achievement of organizational goals and objectives depends on the capabilities of the organization in processing and using the data. However, as the number of data sources increased, and given the technological landscapes. IT characterize today’s IT environment, data integration becomes problematic. This is where the idea of a data integration node is introduced as a models to help one organize the flow of data between nodes.
A data integration node can be described as a point of consolidation where organizations can feed numerous data sources such as databases, applications or any systems for efficient data reconciliation. As organization increases their complexity in using their technologies and infrastructure in undertaking their business processes. This results into the need for having a node in data integration. This paper examines data integration nodes, their advantages, issues involved. The role of these nodes in current complex business environments.
What is a Node in Data Integration?
A data integration node means a specific point in a system. It is network where data are gather, analyze, and disperse to various targets. Essentially it seems like a single pane through which all the information from major systems, database, cloud services or an old application server can be seen in one go. The node allows information from these various contexts to interface with one another and be utilized in a relevant and integrated approach.
A data integration node can be a component of the expanded data integration system. It envisages the control of data processing at an organization. This architecture must include several integration points but the node is an important point. It most of the transformations of data and most of the cleaning and structuring also occur, so data is ready for another use or analytical phase.
Key Benefits of Data Integration Nodes
Enhanced Quality Data Analysis
A data integration node cheques for the quality of data lineage across instances. The data flowing between instances are clean and standardized. Overall, it is able to validate and sanitize data forms and rules. It can prevent or philter out low-quality data from being passed through system interfaces. This enhances the quality of the result data, and thereby providing credible analysis and outcomes for business purposes.
Enhanced Decision-Making
When information is collected from different sources and compiled into one platform, an organization gets a full view of their entity. This fosters better decision making since companies are in a position to look at signals, grasp opportunities and even challenges in their environment using a holistic set of data rather than bits and pieces of information.
Increased Efficiency of Data Integration Node
Importing and exporting data between different systems is a slow process. Most of the time, people end up inputting wrong info. A data integration node handles it automatically, so there is no delay or error in the transfer of data. This frees the employees a lot of time and stress which would have been used in sorting data and statistics.
Scalability of Data Integration Node
This is because as organizations evolve, so does the kinds of data interconnectivity demands that they present. It raises capability of data integration node to be very scalable since additional data to be integrate. This can be obtain from new systems without interrupting the already established integrate data systems. That flexibility also speaks to ease of expansion of business as one does not needs to frequently redesign the database management structures.
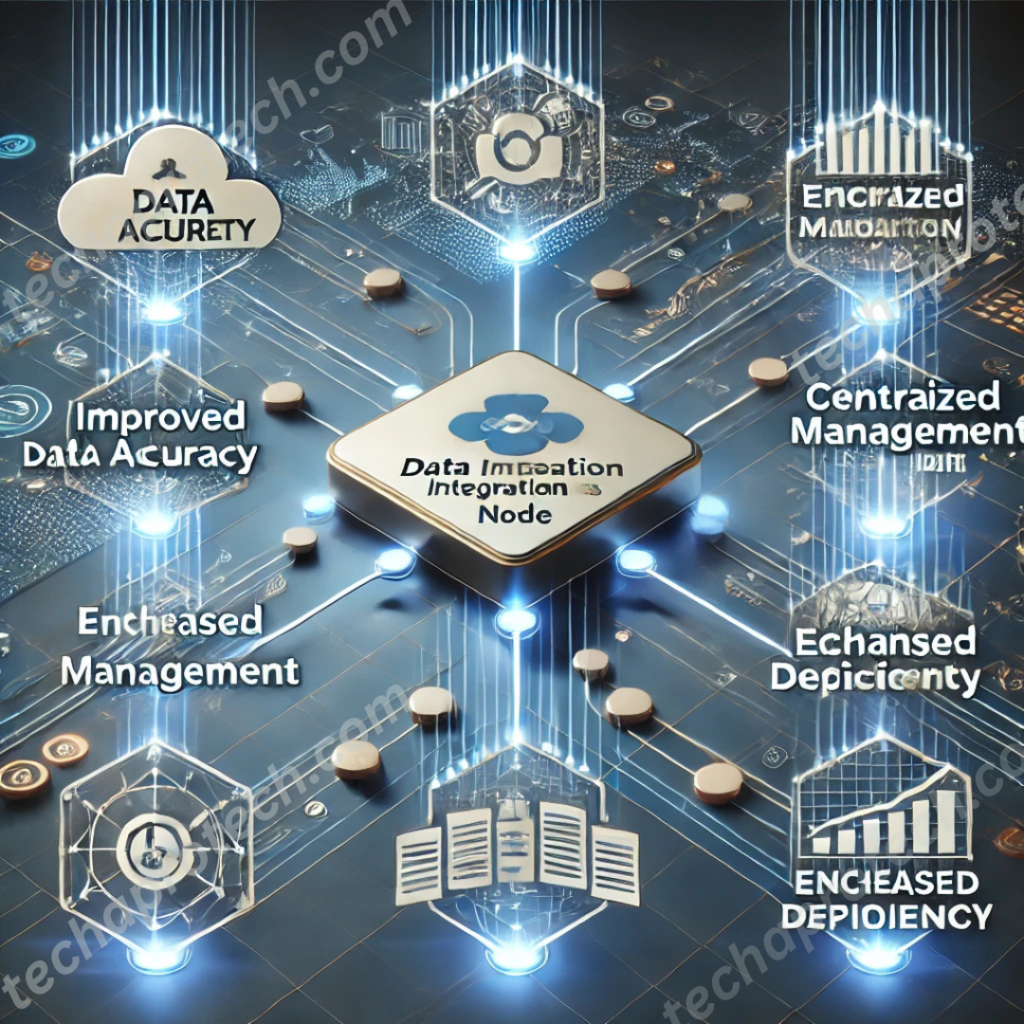
Reflections of the Use of Data Integration Nodes
Similarly, data integration nodes have their own pros but they also present some problems. It is a crucial decision to make for organizations, more so big organizations when it comes to incorporating a data integration node.
Data Security and Privacy of Data Integration Node
Since data transits between two or more systems, security becomes an issue of concern. The process also implies that data integration node has to be protected from unauthorized personnel or a breach. Endpoint security roles and securely integrated pathways also include features sound encapsulation of data, further API protection, and role-based access protocols from a data integration node.
Data Governance of Data Integration Node
So data integration process usually aggregates data from various departments or even from an organization’s partners. It is, therefore, important to ensure that this type of data fulfils governance standards including; ownership, quality and privacy policies. When data governance policies are not implement properly the organization may find themselves in contempt with a law like GDPR or HIPAA.
Legacy Systems Integration
This remains the case with a large number of organizations, as most of them currently employ systems. They were not develop to integrate with modern data integration solutions. Inheriting these older structures into a data integration node could prove to be burdensome, usually calling for the use of special connectors or middle wares. At least as can be decipher from figure two above. With these barriers, it is therefore a question of sacrificing more time, money, or expertise to address the barriers.
Cost and Resource Allocation
Usually, implementing and maintaining a data integration node involves massive investments of both profits and technology. There is, therefore, a need for organizations to dedicate their resources well in software, hardware, and human resource to maximize the performance of the node. To small organizations this can become a big problem.
Industry Guidelines for the Installation of Data Integration Node
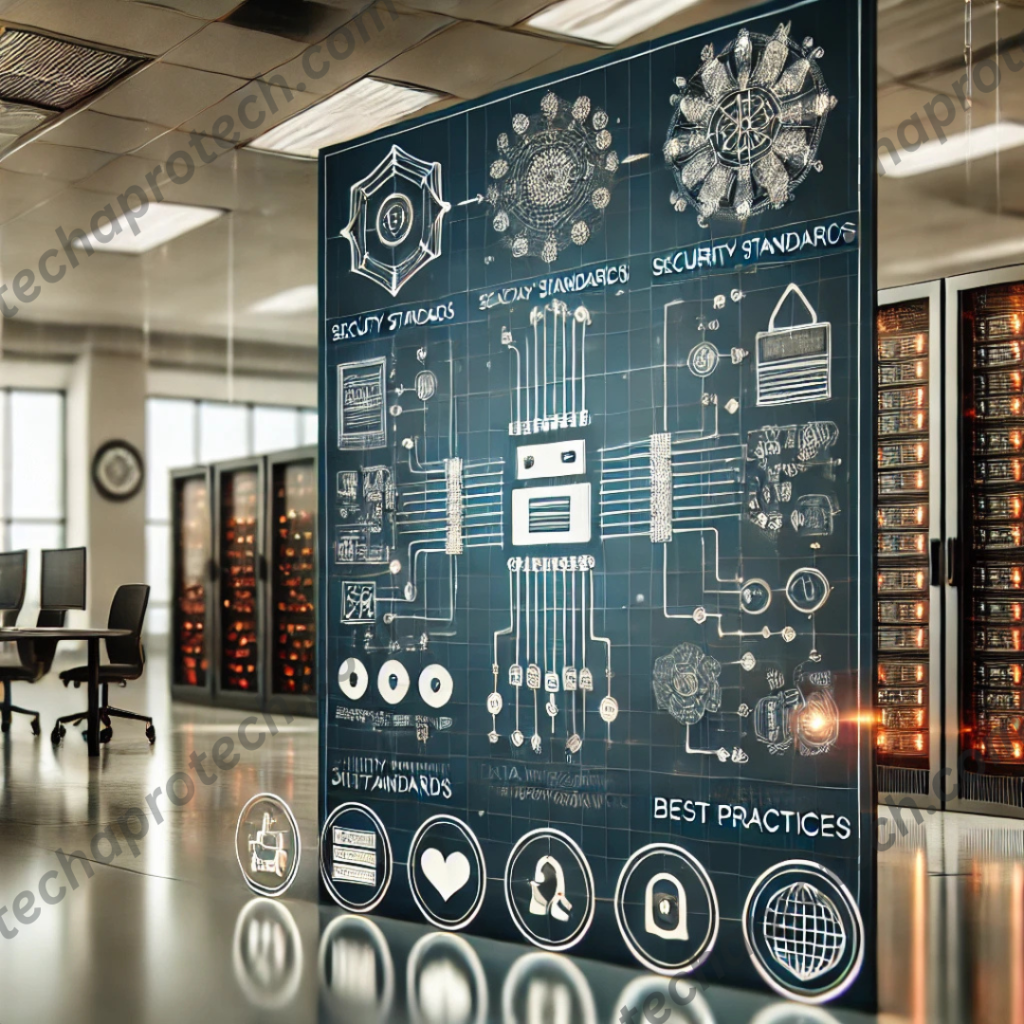
To maximize the benefits of a data integration node, organizations should follow these best practices:
Plan with Scalability in Mind
And as your organization develops, the problems of data integration follow the growth path as well. Propose that your data integration node architecture should have certain extensibility characteristics such that new data sources. Systems and applications that you may decide to incorporate in the architecture later on do not cause significant disruption.
Ensure Data Security of Data Integration Node
Some of the best practices to follow include; Conduct a risk assessment to determine the level of security required to protect your data and then follow the guidelines. Protect each data element by making sure you encrypt your data and place all the necessary access controls on it before collecting the data. Conduct regular audits on the collected data to ensure it’s safety. Given data should be safe therefore your data integration node must meet set industry regulations and standards.
Focus on Data Quality
Keep well defined specifications for validating and cleaning the data. So that the data that is being pull and push during integration procedure in the integration node is good quality. This is because quality data is critical in any integration processes. Any perceived inferiority in the quality of data can be expensive and even compromise the whole integration processes.
Invest in Skilled Personnel
The issues mentioned above prove the fact that a successful data integration project should be accomplished only in case if the IT personnel is skilled and experienced in data architecture, security, and system integration. Just a reminder to have all the expertise necessary for managing and further optimizing the data integration node in your team.
Conclusion
The data integration node is consider as one of the critical components that facilitate effective and efficient data flow within organizations. This is because, as organizations keep on preparing more data from a widening this number of sources, data integration will remain one critical process in business. This paper has demonstrated how a data integration node can significantly support organizations and: Through centralizing data management, improving data quality, and strengthening decision-making. An organization can fully maximize data in an environment that is perpetually data driven.